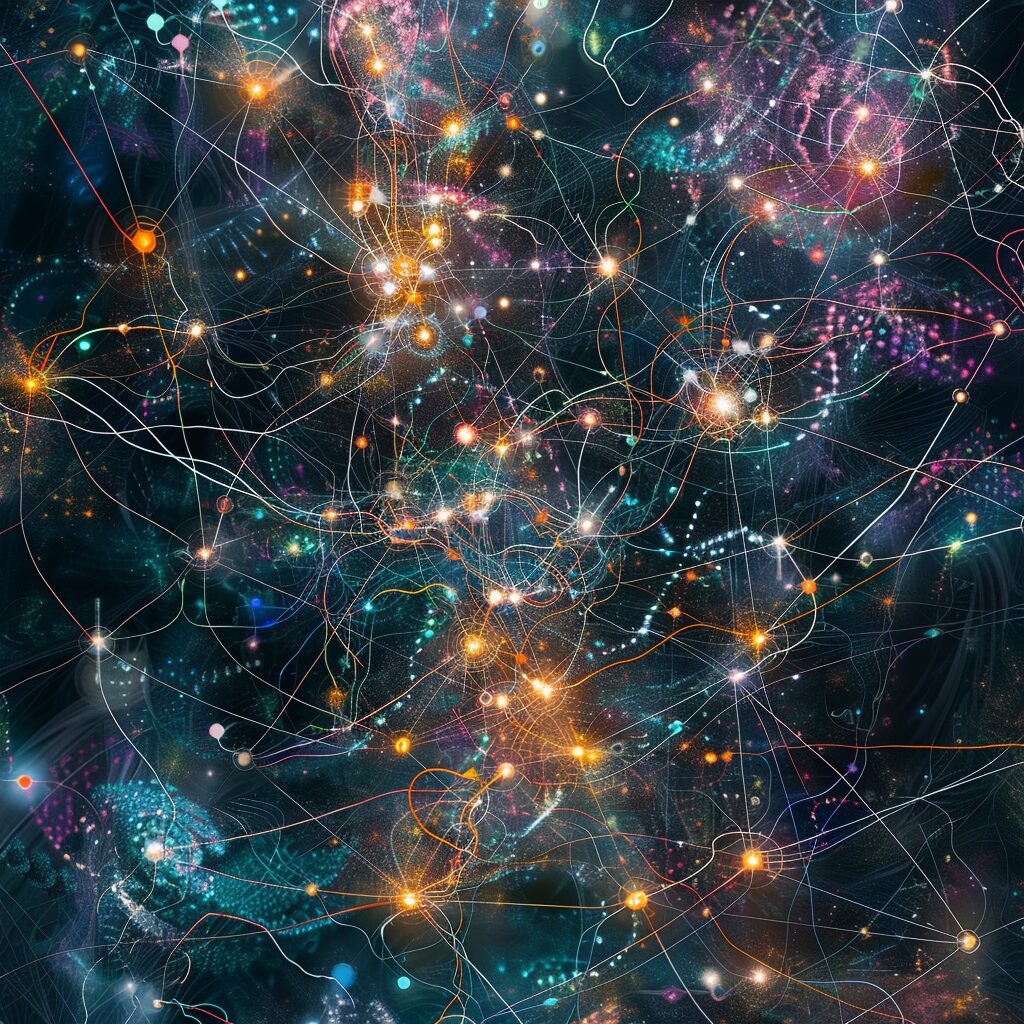
AI in Biophysics: A Revolutionary Catalyst
The integration of artificial intelligence (AI) with biophysics represents a groundbreaking shift in scientific research, catalyzing a new era of discovery and innovation. The extraordinary capabilities of AI—ranging from processing massive datasets to detecting intricate patterns and making highly accurate predictions—are reshaping our approach to understanding biological systems. This fusion of technology and biology is not only refining our grasp of complex phenomena such as protein folding but is also revolutionizing drug discovery and structural biology. As we delve into the various dimensions of this convergence, we will explore how AI is driving transformative advancements in biophysics and discuss the challenges and opportunities that lie ahead.
Protein Folding: A Breakthrough in Biophysical Research
One of the most significant milestones achieved through the application of AI in biophysics is the resolution of the protein folding problem. Proteins are fundamental molecules in biological systems, and their function is intricately linked to their three-dimensional structure. Understanding how proteins fold into their functional forms from their linear amino acid sequences has been a challenge that has persisted for decades. Accurate prediction of protein structures is crucial for comprehending their biological roles and for designing targeted therapies.
The breakthrough in this field was exemplified by AlphaFold, an AI-powered tool developed by DeepMind, a subsidiary of Alphabet Inc. AlphaFold uses deep learning algorithms to predict protein structures with remarkable accuracy. This achievement is based on analyzing extensive databases of protein sequences and structures, allowing the model to learn from vast amounts of existing data. By predicting the 3D structures of proteins with unprecedented precision, AlphaFold has accelerated the pace of research in drug discovery and disease understanding.
For instance, the implications of AlphaFold’s success extend to the study of proteins associated with neurodegenerative diseases such as Alzheimer’s and Parkinson’s. By providing detailed structural insights, AlphaFold facilitates the identification of potential therapeutic targets and the design of novel strategies to combat these debilitating conditions. Additionally, the technology has implications beyond human health; AlphaFold is being employed to engineer enzymes with enhanced capabilities for industrial applications. This includes biofuel production and environmental cleanup, demonstrating the versatile impact of AI-driven protein folding predictions.
Structural Biology: Unveiling the Molecular Blueprint
The impact of AI in structural biology is equally profound. Structural biology aims to decipher the intricate molecular structures of macromolecules—such as proteins and nucleic acids—to understand their functions and interactions. AI-powered image analysis techniques are proving to be invaluable tools in this endeavor, particularly in the analysis of microscopy images.
One of the notable areas where AI is making a difference is in cryo-electron microscopy (cryo-EM). Cryo-EM is a powerful technique that allows researchers to capture high-resolution images of biological macromolecules in their native states. However, interpreting these images can be challenging due to the sheer volume of data and the subtle details involved. AI algorithms, particularly those based on machine learning, can process these images to identify and analyze patterns that might be missed by human experts. This capability enhances our ability to resolve the structures of proteins and protein complexes, leading to new insights into their functions and interactions.
Furthermore, AI is being used to improve the accuracy of image reconstruction and classification in structural biology. Techniques such as convolutional neural networks (CNNs) and other advanced algorithms enable more precise alignment and interpretation of microscopy data. This advancement not only accelerates research but also contributes to a deeper understanding of fundamental biological processes.
Bioinformatics: Revolutionizing Genomic Analysis
In the realm of bioinformatics, AI is transforming how genomic data is analyzed and interpreted. Bioinformatics involves the application of computational techniques to understand biological data, particularly genetic information. With the advent of AI, researchers can now process and analyze genomic data at an unprecedented scale, leading to new discoveries in genetics and personalized medicine.
AI algorithms are employed to identify genetic variations associated with various diseases, including cancer, cardiovascular conditions, and rare genetic disorders. By analyzing large-scale genomic datasets, AI can uncover patterns and correlations that may not be evident through traditional methods. For example, machine learning models can predict which genetic mutations are likely to contribute to cancer development, enabling more accurate risk assessments and personalized treatment plans.
In addition to identifying genetic variations, AI is instrumental in predicting drug responses. By analyzing patients’ genetic profiles and historical treatment data, AI algorithms can forecast how individuals are likely to respond to specific drugs. This capability allows for the development of tailored treatment strategies that are more effective and have fewer adverse effects.
Drug Discovery: Accelerating Therapeutic Innovation
Drug discovery is a field where AI’s impact is particularly transformative. The traditional process of discovering and developing new drugs is often lengthy, expensive, and fraught with uncertainty. AI has the potential to streamline and accelerate this process by identifying promising drug targets, designing novel molecules, and predicting their properties with greater efficiency.
Machine learning algorithms can sift through vast databases of chemical compounds and biological information to identify molecules that have the potential to interact with specific drug targets. This approach not only speeds up the identification of lead compounds but also helps in predicting their efficacy and safety profiles. For instance, AI is being utilized to discover new drug targets for diseases such as malaria and tuberculosis, which have been challenging to address with conventional methods.
AI-driven drug discovery is also enhancing the design of novel drug candidates. By leveraging predictive modeling, researchers can design molecules that are more likely to be effective and have fewer side effects. This approach significantly reduces the time and cost associated with drug development, ultimately leading to faster delivery of new treatments to patients.
Challenges and Opportunities in AI-Driven Biophysics
While the integration of AI into biophysics offers immense potential, it also presents several challenges that need to be addressed. One of the primary concerns is the quality and quantity of data required for training AI models. AI algorithms rely on large datasets to learn and make accurate predictions. Ensuring that these datasets are comprehensive, accurate, and representative is crucial for the reliability of AI-driven insights.
Another challenge is the potential for bias in AI algorithms. Biases in data or algorithmic design can lead to skewed results and inequitable outcomes. Addressing these biases requires careful consideration of data sources, algorithmic transparency, and ongoing evaluation of model performance.
Ethical considerations also play a significant role in the application of AI in biophysics. The use of patient data, for instance, necessitates stringent privacy measures to protect individuals’ sensitive information. Researchers and practitioners must adhere to ethical guidelines that ensure the responsible use of AI technologies and uphold the principles of data privacy and security.
Despite these challenges, the opportunities presented by AI in biophysics are substantial. By developing robust data management practices and ethical frameworks, researchers can maximize the benefits of AI while mitigating potential risks. Collaborative efforts between AI experts, biophysicists, and ethicists will be essential in navigating these challenges and ensuring that AI continues to advance our understanding of biological systems in a responsible and effective manner.
Conclusion
The integration of AI into biophysics represents a revolutionary leap in scientific research, offering unprecedented capabilities in data analysis, pattern recognition, and prediction. From solving the protein folding problem to accelerating drug discovery and enhancing structural biology, AI is driving significant advancements across the field. As AI technologies continue to evolve, they promise to unlock even greater insights into biological systems and contribute to the development of innovative therapeutic strategies.
The ongoing convergence of AI and biophysics is a testament to the transformative power of interdisciplinary collaboration. By addressing the challenges associated with data quality, algorithmic bias, and ethical considerations, researchers can harness the full potential of AI to push the boundaries of scientific knowledge and improve human health. As we move forward, the continued exploration of AI-driven biophysics will undoubtedly lead to exciting new discoveries and innovations that will shape the future of both fields.
Additional Insights
- AI-Driven Drug Repurposing: AI can be used to identify existing drugs that might be effective for new diseases, accelerating drug discovery and reducing development costs.
- AI-Powered Microscopy: AI is being used to develop new microscopy techniques, such as super-resolution microscopy, that allow researchers to visualize biological structures at the nanoscale.
- AI for Personalized Medicine: AI can be used to analyze patient data and develop personalized treatment plans based on individual genetic profiles and medical history.
- AI for Synthetic Biology: AI is being used to design and optimize synthetic biological systems, such as engineered bacteria for producing biofuels or pharmaceuticals.
- AI for Understanding Complex Biological Systems: AI can help researchers to understand complex biological systems, such as the human brain, by analyzing large-scale datasets and identifying patterns and relationships.
References:
Arrieta, A. B., Díaz-Rodríguez, N., Ser, J., Bennetot, A., Tabik, S., Barbado, A., … & Herrera, F. (2019). Explainable Artificial Intelligence (XAI): Concepts, Taxonomies, Opportunities and Challenges toward Responsible AI. Information Fusion, 58, 82-115.
Tsuboyama, K., Dauparas, J., Chen, J., Laine, É., Behbahani, Y. M., Weinstein, J. J., … & Rocklin, G. (2023). Mega-scale experimental analysis of protein folding stability in biology and design. Nature, 620, 434-444.
Lundberg, S.M., Erion, G.G., Chen, H., DeGrave, A.J., Prutkin, J.M., Nair, B.G., Katz, R., Himmelfarb, J., Bansal, N., & Lee, S. (2020). From local explanations to global understanding with explainable AI for trees. Nature Machine Intelligence, 2, 56 – 67.
Chai, C., Wang, J., Luo, Y., Niu, Z., & Li, G. (2023). Data Management for Machine Learning: A Survey. IEEE Transactions on Knowledge and Data Engineering, 35, 4646-4667.
Zhang, D., Gao, F., Jakovlić, I., Zhou, H., Zhang, J., Li, W.X., & Wang, G. (2019). PhyloSuite: An integrated and scalable desktop platform for streamlined molecular sequence data management and evolutionary phylogenetics studies. Molecular Ecology Resources, 20, 348 – 355.
Dave, T., Athaluri, S.A., & Singh, S. (2023). ChatGPT in medicine: an overview of its applications, advantages, limitations, future prospects, and ethical considerations. Frontiers in Artificial Intelligence, 6.
Huber, W., Carey, V.J., Gentleman, R., Anders, S., Carlson, M., Carvalho, B.S., Bravo, H.C., Davis, S.R., Gatto, L., Girke, T., Gottardo, R., Hahne, F., Hansen, K.D., Irizarry, R.A., Lawrence, M., Love, M.I., MacDonald, J., Obenchain, V., Oles, A.K., Pagès, H., Reyes, A., Shannon, P.T., Smyth, G.K., Tenenbaum, D., Waldron, L., & Morgan, M.T. (2015). Orchestrating high-throughput genomic analysis with Bioconductor. Nature Methods, 12, 115-121.
Chen, R.J., Lu, M.Y., Williamson, D.F., Chen, T.Y., Lipková, J., Noor, Z., Shaban, M., Shady, M., Williams, M., Joo, B., & Mahmood, F. (2022). Pan-cancer integrative histology-genomic analysis via multimodal deep learning. Cancer cell, 40 8, 865-878.e6 .